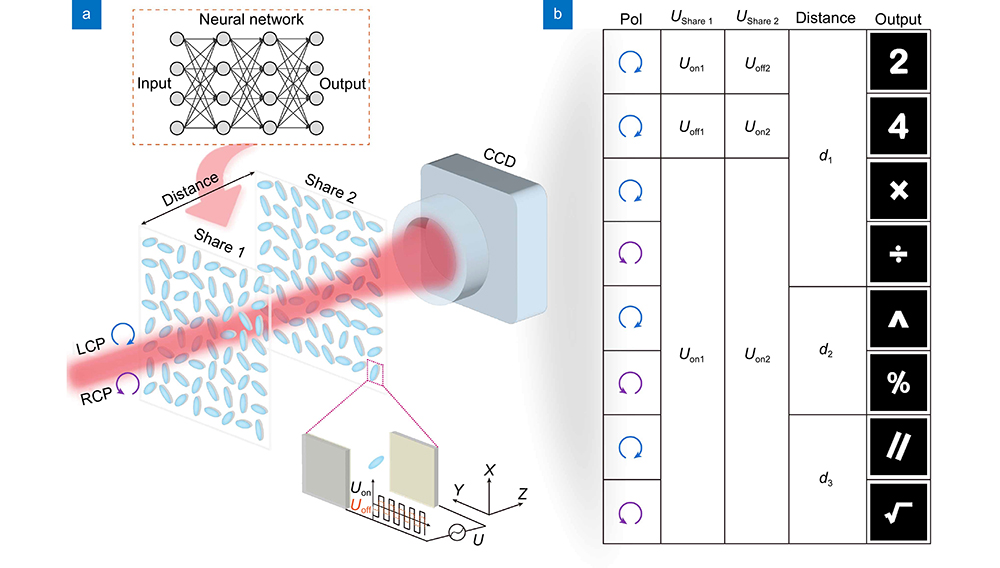
Author Affiliations
Abstract
1 Institute of Photonic Chips, University of Shanghai for Science and Technology, Shanghai 200093, China
2 Centre for Artificial-Intelligence Nanophotonics, School of Optical-Electrical and Computer Engineering, University of Shanghai for Science and Technology, Shanghai 200093, China
3 National Laboratory of Solid State Microstructures, and College of Engineering and Applied Sciences, Nanjing University, Nanjing 210093, China
Secret sharing is a promising technology for information encryption by splitting the secret information into different shares. However, the traditional scheme suffers from information leakage in decryption process since the amount of available information channels is limited. Herein, we propose and demonstrate an optical secret sharing framework based on the multi-dimensional multiplexing liquid crystal (LC) holograms. The LC holograms are used as spatially separated shares to carry secret images. The polarization of the incident light and the distance between different shares are served as secret keys, which can significantly improve the information security and capacity. Besides, the decryption condition is also restricted by the applied external voltage due to the variant diffraction efficiency, which further increases the information security. In implementation, an artificial neural network (ANN) model is developed to carefully design the phase distribution of each LC hologram. With the advantage of high security, high capacity and simple configuration, our optical secret sharing framework has great potentials in optical encryption and dynamic holographic display.
holographic encryption optical secret sharing cascaded liquid crystal hologram multi-dimensional multiplexing Opto-Electronic Advances
2024, 7(1): 230121

Author Affiliations
Abstract
1 Institute of Photonic Chips, University of Shanghai for Science and Technology, Shanghai 200093, China
2 School of Optical-Electrical and Computer Engineering, University of Shanghai for Science and Technology, Shanghai 200093, China
3 Nokia Shanghai Bell Co., Ltd., Shanghai 201206, China
4 College of Medical Instruments, Shanghai University of Medicine and Health Sciences, Shanghai 201318, China
Edge detection for low-contrast phase objects cannot be performed directly by the spatial difference of intensity distribution. In this work, an all-optical diffractive neural network (DPENet) based on the differential interference contrast principle to detect the edges of phase objects in an all-optical manner is proposed. Edge information is encoded into an interference light field by dual Wollaston prisms without lenses and light-speed processed by the diffractive neural network to obtain the scale-adjustable edges. Simulation results show that DPENet achieves F-scores of 0.9308 (MNIST) and 0.9352 (NIST) and enables real-time edge detection of biological cells, achieving an F-score of 0.7462.
diffractive neural network edge detection phase objects Chinese Optics Letters
2024, 22(1): 011102
1 上海理工大学光电信息与计算机工程学院, 上海 200093
2 上海理工大学光电信息与计算机工程学院, 上海 200093栾
相位定量检测是检测入射光场波前的重要指标, Shack-Hartmann波前传感器能够同时测量波前的振幅和相位分布, 但其分辨率较低。针对传统的非干涉相位检测法需要两台放置在不同位置的相机, 使用两幅图像之间的相位差来估算波前的相位信息, 造成了相位成像系统的复杂性和不确定性的问题, 提出了将宽带光源作为照明光源, 只需单次拍摄彩色图像输入神经网络并使用梯度下降算法进行优化的相位重建方法。仿真和试验结果表明, 这项技术将物理模型和神经网络相结合, 能够以高分辨、高速度、低成本实现待测物体的相位重建工作。其中作为评价重建图像质量的均方误差函数(MSE)最小可达到0.089 rad, 对于透明细胞的相位重建质量为0.133 rad, 要优于GS法(0.320 rad)和TIE法(0.378 rad)。因此, 提出的基于神经网络的宽带光源相位重建方法可以运用于自适应光学、活细胞生物实时成像等领域。
非相干检测 相位重建 神经网络 梯度下降算法 incoherent detection phase retrieval neural network gradient descent algorithm

Author Affiliations
Abstract
The orbital angular momentum (OAM) of light has been implemented as an information carrier in OAM holography. Holographic information can be multiplexed in theoretical unbounded OAM channels, promoting the applications of optically addressable dynamic display and high-security optical encryption. However, the frame-rate of the dynamic extraction of the information reconstruction process in OAM holography is physically determined by the switching speed of the incident OAM states, which is currently below 30 Hz limited by refreshing rate of the phase-modulation spatial light modulator (SLM). Here, based on a cross convolution with the spatial frequency of the OAM-multiplexing hologram, the spatial frequencies of an elaborately-designed amplitude distribution, namely amplitude decoding key, has been adopted for the extraction of three-dimensional holographic information encoded in a specific OAM information channel. We experimentally demonstrated a dynamic extraction frame rate of 100 Hz from an OAM multiplexing hologram with 10 information channels indicated by individual OAM values from –50 to 50. The new concept of cross convolution theorem can even provide the potential of parallel reproduction and distribution of information encoded in many OAM channels at various positions which boosts the capacity of information processing far beyond the traditional decoding methods. Thus, our results provide a holographic paradigm for high-speed 3D information processing, paving an unprecedented way to achieve the high-capacity short-range optical communication system.
Opto-Electronic Science
2022, 1(9): 220004
1 上海理工大学光子芯片研究院, 上海 200093
2 上海理工大学光电信息与计算机工程学院人工智能纳米光子学中心, 上海 200093
完美涡旋光束 (POVB) 是径向强度分布和半径均与光束轨道角动量 (OAM) 状态无关的一类涡旋光, 已被应用于光学操控、光通信、激光材料处理等领域。其中, POVB 轨道角动量状态的探测是关键且有挑战的技术。本研究通过并行梯度下降算法, 构建了光学衍射神经网络 (DNN), 实验上实现了轨道角动量阶数在-50~+50 范围内的 POVB 的识别。在此过程中, 衍射转换效率可达 58%。本研究为 POVB 的 OAM 探测提供了新的思路, 在 POVB 的各类应用中均存在潜在应用价值。
傅里叶光学 轨道角动量探测 光学衍射神经网络 完美涡旋光束 Fourier optics orbital angular momentum detection optical diffraction neural network perfect optical vortex beam

Author Affiliations
Abstract
1 University of Shanghai for Science and Technology, Institute of Photonic Chips, Shanghai, China
2 University of Shanghai for Science and Technology, School of Optical-Electrical and Computer Engineering, Centre for Artificial-Intelligence Nanophotonics, Shanghai, China
The creation of biomimetic neuron interfaces (BNIs) has become imperative for different research fields from neural science to artificial intelligence. BNIs are two-dimensional or three-dimensional (3D) artificial interfaces mimicking the geometrical and functional characteristics of biological neural networks to rebuild, understand, and improve neuronal functions. The study of BNI holds the key for curing neuron disorder diseases and creating innovative artificial neural networks (ANNs). To achieve these goals, 3D direct laser writing (DLW) has proven to be a powerful method for BNI with complex geometries. However, the need for scaled-up, high speed fabrication of BNI demands the integration of DLW techniques with ANNs. ANNs, computing algorithms inspired by biological neurons, have shown their unprecedented ability to improve efficiency in data processing. The integration of ANNs and DLW techniques promises an innovative pathway for efficient fabrication of large-scale BNI and can also inspire the design and optimization of novel BNI for ANNs. This perspective reviews advances in DLW of BNI and discusses the role of ANNs in the design and fabrication of BNI.
direct laser writing neuron interface neural tissue engineering artificial neural networks Advanced Photonics
2022, 4(3): 034002
1 上海理工大学光子芯片研究院, 上海 200093
2 上海理工大学光电信息与计算机工程学院人工智能纳米光子学中心, 上海 200093
人工智能技术,特别是人工神经网络的创新引领了许多领域的应用革命,如网络搜索、计算机识别和语言、图像的识别技术。近年来纳米光子学的发展为传统的人工神经网络技术,特别是光学神经网络的发展带来了全新的物理视角以及截然不同的实现方法。一方面,纳米光子学是一门研究光与材料在纳米尺度相互作用的科学,可以带来全新的技术,如超分辨光学加工技术和超分辨光学成像技术,进而推动微纳尺度上多种功能的光学神经网络的实现。另一方面,纳米光子学中光子传播的多频段、高速度、低功耗的特点,促使了光学神经网络向着小体积、高密度、低功耗的方向发展。人工神经网络自身的发展也促使神经网络算法(如逆向设计、深度学习)在纳米光子学器件的设计中发挥前所未有的作用,以满足纳米光子学器件对自身功能、体积、集成度、计算功能的日益增长的要求。以神经网络的发展为起点,阐述人工神经网络特别是光学神经网络的发展趋势,以及人工神经网络与纳米光子学相互促进的发展历程。
光学器件 人工智能 人工神经网络 光学神经网络 纳米光子学 光学人工智能
Author Affiliations
Abstract
1 Laboratory of Artificial-Intelligence Nanophotonics, School of Science, RMIT University, Melbourne, Victoria 3001, Australia
2 Centre for Artificial-Intelligence Nanophotonics, School of Optical-Electrical and Computer Engineering, University of Shanghai for Science and Technology, Shanghai 200093, China
Neuromorphic computing applies concepts extracted from neuroscience to develop devices shaped like neural systems and achieve brain-like capacity and efficiency. In this way, neuromorphic machines, able to learn from the surrounding environment to deduce abstract concepts and to make decisions, promise to start a technological revolution transforming our society and our life. Current electronic implementations of neuromorphic architectures are still far from competing with their biological counterparts in terms of real-time information-processing capabilities, packing density and energy efficiency. A solution to this impasse is represented by the application of photonic principles to the neuromorphic domain creating in this way the field of neuromorphic photonics. This new field combines the advantages of photonics and neuromorphic architectures to build systems with high efficiency, high interconnectivity and high information density, and paves the way to ultrafast, power efficient and low cost and complex signal processing. In this Perspective, we review the rapid development of the neuromorphic computing field both in the electronic and in the photonic domain focusing on the role and the applications of memristors. We discuss the need and the possibility to conceive a photonic memristor and we offer a positive outlook on the challenges and opportunities for the ambitious goal of realising the next generation of full-optical neuromorphic hardware.